Explainable AI: Challenges And Opportunities In Developing Transparent Machine Learning Models
25 May 2023
One of the biggest problems with artificial intelligence (AI) is that it’s very difficult for us to understand how it works – it’s just too complicated!
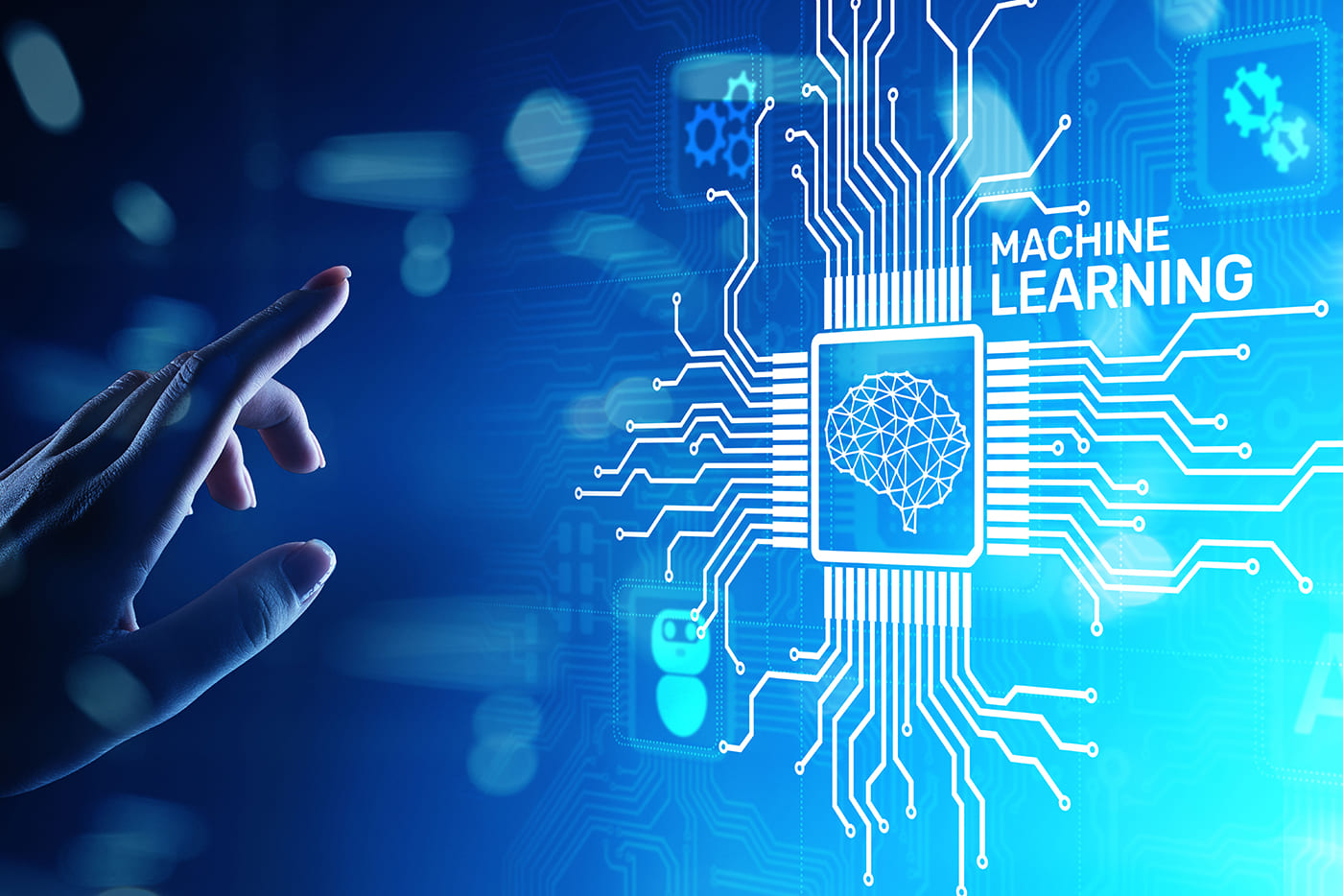
Take ChatGPT – the chatbot that has become a viral sensation in recent months – as an example. It’s capable of generating written text such as emails, stories, blog posts, and poems to such a high standard that they appear to be written by a human.
It also makes some very silly mistakes and sometimes talks plain nonsense. And because the algorithms that produce its output are so complex (the latest iteration of its language model, known as GPT-4, is said to be trained on over one trillion parameters), no one is really sure why. Where is it going wrong? And what is the root cause of the mistakes it makes?
This is what gives rise to the challenge of creating “explainable AI” (XAI). This is a term for AI systems that go beyond giving us an answer to the questions we ask them. An XAI system should be able to give us clear, easily understandable explanations for its decisions and details of the factors it has taken into account when making them.
Think back to when you were at school, and the teacher would expect you to "show your workings" – so that they’d know you understood your answer and hadn't just guessed or copied it from the child sitting at the next desk. AI needs to be explainable for the same reason your schoolwork was!
So, let’s take a look at some of the reasons this is essential to the future development of AI and a major challenge we need to solve if AI is going to live up to its promised potential.
Why is Explainable AI Vital?
AI has the potential to revolutionize industries from healthcare to finance. In order to do so, though, we need to be able to trust it. Not just that – we need to be super-confident that we understand why it is recommending a patient receive a particular treatment or how it knows that an incoming multi-million-dollar transaction is highly likely to be fraudulent.
AI algorithms can only give answers that are as good as the data they've been trained on. If they've been trained on incorrect or biased data, then the answers will be incorrect. If we're expecting to use it to make important decisions that could affect people's lives, for example, on issues of healthcare, employment, or finance, this could be dangerous and very bad for society in general.
An old saying about computer algorithms and data processing is "garbage in = garbage out," and this is doubly true for AI algorithms.
At the end of the day, it all boils down to trust – AI has the potential to transform society and improve our lives in some pretty amazing ways, but that will only happen if society can trust it.
Solving the “black box problem” – creating explainable AI – is a vital part of achieving this because people (in general) are far more likely to feel that they can trust AI and feel comfortable allowing it to use their data and make decisions, if they can understand it.
Explainable AI is also an important concept from a regulatory point of view. As AI becomes more ingrained in society, it’s likely that more laws and regulations will appear, governing its use. An example of this is European Union's AI Act. Whether or not an application makes itself explainable may well play an important role in determining how it is regulated in the future.
Challenges of Developing Explainable AI
The first challenge comes about due to the complexity of AI itself. When we talk about AI today, we generally mean machine learning. This refers to algorithms that can become better and better at a particular task - from recognizing images to navigating an autonomous vehicle – as they are fed more and more data. This requires complex, mathematical models which are difficult to translate into explanations that are simple for humans to understand.
Another issue is that explainability requires a trade-off with performance – most machine learning algorithms are coded in such a way that they will provide a result as efficiently as possible, without expending resources on explaining what they are doing.
And there are also commercial concerns at play. Details of the exact workings of some of the most widely used machine learning systems – such as Google’s search algorithms or ChatGPT’s language model – are not made publicly available. One reason for this is that it would make it easy for competitors to copy them and undermine the commercial advantage of their owners.
How Are These Challenges Being Tackled?
Solving the challenges of XAI is likely to require widespread and ongoing collaboration between all stakeholder organizations. This includes the academic and research organizations where new developments are made, the commercial entities that make the technology available and use it to generate profits and governmental bodies that will play a role in regulating and overseeing its adoption by society.
IBM, for example, has created an open-source toolkit called Ai Explainability 360 that can be used by AI developers to build concepts of explainability into their projects and applications.
Many academic institutions, non-governmental organizations as well as private companies have established their own research institutions focused on ethical AI, and transparency is often a focus of their research.
One priority is establishing standardized benchmarks and metrics that can be used to measure explainability – which today could mean different things to different people. Agreeing on how it can be assessed and how applications and projects that allow a good level of explainability can be promoted for wider adoption is an important part of this work.
Could AI Itself Provide The Answer?
Natural language tools like ChatGPT have already shown that they are capable of annotating computer code so that it explains what it’s doing in human language. It’s likely that future iterations of this technology will be sophisticated enough that they can annotate AI algorithms as well.
When the GPT-3 and GPT-4 language models that power ChatGPT were integrated into Microsoft’s Bing search engine, functionality was added that shows (to a limited extent) where the algorithms are finding the data that are used to provide answers to users' queries. This is a step forward in terms of providing explainability – certainly when compared to the original ChatGPT application, which offers no clue or explanation at all.
Whatever solutions are put into place, we can say with confidence that the quest to provide XAI will play an important role in preparing society for the changes that AI, in general, is set to bring about. As AI plays an increasingly prominent role in our lives, it will encourage developers of AI tools and applications to adopt responsible and ethical practices in pursuit of trust and transparency. This, in turn, will hopefully lead us toward a future where AI is used in a way that’s fair and beneficial to us all.
Related Articles
The Simple ChatGPT Trick That Will Transform Your Business AI Interactions
I believe ChatGPT and other generative AI tools can help pretty much any business.[...]
The Third Wave Of AI Is Here: Why Agentic AI Will Transform The Way We Work
The chess pieces of artificial intelligence are being dramatically rearranged. While previous iterations of AI focused on making predictions or generating content, we're now witnessing the emergence of something far more sophisticated: AI agents that can independently perform complex tasks and make decisions.[...]
How Generative AI Will Change Jobs In Cybersecurity
Ensuring robust cybersecurity measures are in place is more important than ever when it comes to protecting organizations and even governments and nations from digital threats.[...]
The 10 Most Important Banking And Financial Technology Trends That Will Shape 2025
As technological disruption and economic uncertainty continue to reshape the financial landscape, alongside dramatic shifts in consumer behavior and regulatory requirements, 2025 promises to be both challenging and opportunistic for banking and financial services.[...]
The 6 Most Powerful AI Marketing Trends That Will Transform Your Business In 2025
The quiet hum of AI servers is rapidly drowning out the traditional drumbeat of marketing departments worldwide.[...]
AI Everywhere – Scaling AI In The Cloud With Intel® Xeon®6
Today, the omnipresent AI that we’re starting to take for granted has become a critical tool for business.[...]
Sign up to Stay in Touch!
Bernard Marr is a world-renowned futurist, influencer and thought leader in the fields of business and technology, with a passion for using technology for the good of humanity.
He is a best-selling author of over 20 books, writes a regular column for Forbes and advises and coaches many of the world’s best-known organisations.
He has a combined following of 4 million people across his social media channels and newsletters and was ranked by LinkedIn as one of the top 5 business influencers in the world.
Bernard’s latest book is ‘Generative AI in Practice’.
Social Media